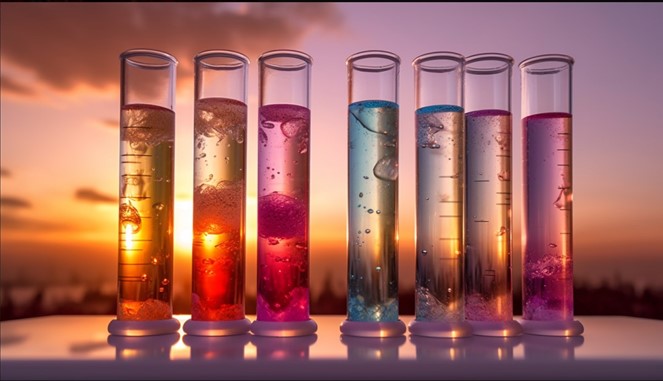
Enabling Better Drug Discovery Outcomes with Machine Learning
Can the long road to bring new medical treatments or therapies to market be shortened by introducing artificial intelligence? AI applied to the early stage of the discovery process, which often involves new insight into a disease or treatment mechanism, may soon provide researchers many more potential candidates or designs to evaluate. AI can also help in the sorting and evaluation of these candidates to improve the success rates of those that make it into the lab for further study.
Benefits AI Brings to Biotech Research
The cost of bringing a single drug to market in terms of time and money is substantial. Estimates are in the $2.8 billion range, and the average timeline for drug development exceeds a decade. On top of this, there is a low level of certainty of taking a promising molecule all the way to market. The success rate of translating preclinical research findings into effective clinical treatments is low; failure rates are estimated to be around 90%.
The refinement of digital sorting and calculating with advanced computational technologies, such as artificial intelligence (AI) and machine learning (ML), have the potential to revolutionize pharmaceutical research and development (R&D). Despite it still being a young technology, AI-enabled applications and algorithms are already making an impact in drug discovery and development processes.
One of the significant benefits of ML in drug development is its ability to recognize patterns and unveil insights that might be missed by conventional data analysis or take substantially less time to recognize. AI, and ML technologies can help a biotech company do precursory evaluation, accelerate the design and testing of molecules, streamline the testing processes, and provide a faster understanding along the way if the molecule will perform as expected. With improved clinical success and reduced costs throughout the development pipeline, AI may be shot in the arm the industry needs.
Adoption of AI in Biotechnology
While any full-scale adoption of AI in the pharmaceutical industry is still evolving and finding its place, implementation and investment are growing. Top global pharmaceutical companies have increased their R&D investment in AI by nearly 25% over the past three years – this indicates a recognition of the perceived benefits.
The interest and investment in AI drug discovery is fueled by several factors. As touched on earlier, a more efficient and cost-effective drug development process would be of great benefit. AI can significantly reduce both time and cost. And the sooner more effective treatments are available, the better. Chronic diseases, such as cancer, autoimmune problems, neurological disorders, and cardiovascular diseases, creates an ongoing demand for improved drugs and therapies. AI’s ability to analyze vast amounts of data, identify patterns, and then learn from the information at an accelerated rate can allow researchers to shorten timelines to final conclusions.
Even more exciting is the growing availability of large datasets thanks to the rise of big data. With an increase in the volume, variety and velocity of data, and the AI-assisted ability to make sense of it, outcomes are expected to be improved. These datasets, obtained from various sources like electronic medical records and genomic databases, allow successful AI applications in drug discovery. Technological advancements, especially in ML algorithms, have been contributing to the growth of AI in medicine. And they are growing more sophisticated, allowing for accurate pattern identification in complex biological systems. Collaborations between academia, industry, and government agencies have further accelerated growth sharing knowledge and resources.
Trends in AI and ML Biotechnology
While considered a young technological field, AI-enabled drug discovery is being shaped by a number of new trends and technologies. Modern AI algorithms are now capable of analyzing intricate biological systems and foretelling the effects of medications on human cells and tissues. By detecting probable adverse effects early on in the development phase, the predictive ability helps prevent failures in the later stages.
By generating candidates that fit certain requirements, generative models can accelerate the design of completely new medications. But other technology is also now available to assist. By offering scalable processing resources, cloud computing dramatically cuts down on both time and expense. By simulating the interaction of hundreds of chemicals with disease targets, virtual drug screening enables the fast screening of drugs.
A higher understanding of disease biology and the discovery of new therapeutic targets is being made possible by integrative techniques that incorporate many data sources not available a short while ago.
Constraints on AI-Assisted Biotech Research
While AI can speed up certain aspects of drug discovery, it cannot replace most traditional lab testing. Hands-on experimentation and data collection on living organisms are expected to always be necessary, many of these processes during the clinical trial stages cannot be sped up.
Regulatory bodies, like the FDA, are also cautious about embracing AI fully, raising concerns about transparency and accountability in decision-making processes.
.
Take Away
The near future of artificial intelligence and machine learning assuming a larger role in enabling drug discovery and more efficient R&D looks bright. The technology offers real promise for more efficient and cost-effective drug development processes – this would address the need for new therapies for chronic diseases.
The time-consuming process of testing on real subjects is not expected to be replaced or overly streamlined by technology, but finding subjects and evaluating results can also benefit from the new technology.
Managing Editor, Channelchek
Sources
https://www.fda.gov/patients/learn-about-drug-and-device-approvals/drug-development-process